Joshua Schrier
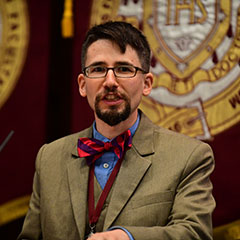
Professor of Chemistry
Email: [email protected]
Office: JMH 616
Lab: JMH 620
Phone: 718-817-4453
Linked-In Profile
-
- Professor at Fordham University (2018-)
- Kim B. And Stephen E. Bepler Chair in Chemistry at Fordham University (2018-)
- Department chair at Haverford College 2017-2018
- Associate professor at Haverford College 2015-2018
- Assistant profesor at Haverford College 2008-2015
- Luis W. Alvarez Postdoctoral Fellow at Lawrence Berkeley National Laboratory (2005-2008)
- Ph.D. in Chemistry (Physical/Theoretical) from University of California, Berkeley
- B. Sc. In Chemistry and Biochemistry (summa cum laude) from St. Peter's College
Joshua A. Schrier comes to Fordham from Haverford College, where he has amassed an impressive record of research into the computational design of new materials for use in information and energy technology. He has authored 44 peer-reviewed papers, most of which were published with his undergraduate students. His research lies at the intersection of quantum mechanics, chemistry, and computational science, with bearing on low-cost electronics, photovoltaics, and batteries needed for the widespread adoption of renewable energy.
While at Haverford, he received a prestigious Henry Dreyfus Teacher-Scholar Award, served as a Fulbright scholar at the Fritz Haber Institute of the Max Planck Society, and obtained more than $8.6 million in external funding. He earned his doctorate from the University of California, Berkeley, in 2005 and served as Luis W. Alvarez postdoctoral fellow at the Lawrence Berkeley National Laboratory before joining Haverford College in 2008. In 2017, Professor Schrier published a textbook focused on applying modern computational techniques to the chemistry curriculum.
-
My main interest is in using computers to accelerate the discovery of new materials, by using a combination of physics-based simulations, cheminformatics, machine learning, and automated experimentation. The promises and challenges of these efforts have been summarized in recent workshop reports by NIST and DOE, the Mission Innovation initiative, and the NSF. More generally, I am interested in the integration of modern computational techniques into the chemistry curriculum at all levels.
I am currently leading a multi-institutional effort to develop a robotic system—capable of performing the equivalent of a year of human experimentation in less than a week of operation—for the discovery of new organohalide perovskite materials. This project was highlighted at the 2019 DARPA AI Colloquium. Capturing a comprehensive description of the experiment in machine readable form allows us to apply machine learning methods to accelerate the discovery of new materials and extract new thermodynamic rules for perovskite formation, and provide comprehensive, reproducible and A second effort is an initiative to harness the unpublished failures and marginal successes in laboratory notebooks ("dark reactions"), turn them into digital data, and use that to advance hydrothermal synthesis of templated metal oxide materials. A subproject within this effort is the role human factors in scientific decision making, and development of methods to improve the quality of experiment plans.
-
Please see Google Scholar for a complete list of publications.
(* denotes undergraduate student co-authors)J. Schrier, Introduction to Computational Physical Chemistry (Mill Valley, California: University Science Books, 2017) 505pp. http://www.uscibooks.com/schrier.htm
X. Jia*, A. Lynch*, Y. Huang*, M. Danielson*, I. Lang’at*, A. Milder*, A. E. Ruby*, H. Wang*, S. A. Friedler, A. J. Norquist, J. Schrier, “Anthropogenic biases in chemical reaction data hinder exploratory inorganic synthesis” Nature (in press, 2019)
Z. Li, M. Ani Najeeb, L. Alves*, A. Z. Sherman*, P. Cruz Parilla*, I. M. Pendleton, M. Zeller, J. Schrier, A. J. Norquist, E. M. Chan, "Robot-Accelerated Perovskite Investigation and Discovery (RAPID): 1. Inverse Temperature Crystallization" (submitted, 2019)
I. M. Pendleton, G. Cattabriga, Z. Li, M. Ani Najeeb, S. A. Friedler, A. J. Norquist, E. Chan, J. Schrier, “Experiment Specification, Capture and Laboratory Automation Technology (ESCALATE): A software pipeline for automated chemical experimentation and data management” MRS Communications (published online June 2019) doi:10.1557/mrc.2019.72
P. Raccuglia*, K. C. Elbert*, P. D. F. Adler, C. Falk*, M. B. Wenny*, A. Mollo*, M. Zeller, S. A. Friedler, J. Schrier, A. J. Norquist, “Machine learning assisted materials discovery using failed experiments” Nature 533, 73-76 (2016) doi:10.1038/nature17439