Frank Hsu
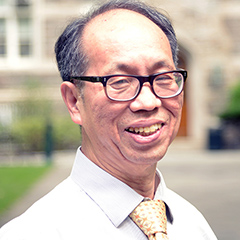
Clavius Distinguished Professor
Fordham University
Department of Computer and Information Sciences
John Mulcahy Hall - 402B
Email: [email protected]
Phone: 718-817-4483
-
D. Frank Hsu is the Clavius Distinguished Professor of Science, a Professor of Computer and Information Science(CIS), and the Director of Fordham Laboratory of Informatics and Data Mining (LIDM) at Fordham University. He received a B.A. degree from Cheng Kung University, an M.A. degree from the University of Texas at El Paso (UTEP), and a Ph.D. degree from the University of Michigan.
At Fordham, Dr. Hsu served as Chair of the CIS Dept. twice (1987-1992, 1999-2006) and was Associate Dean of the Graduate School of Arts and Sciences (2008-2009). He co-organized the Fordham-IBM Forum on Analytics (2012) and the Fordham-IBM Workshop on Blockchain (2017). He co-founded the International Conference on Cyber Security (ICCS) in 2009 and is ongoing at Fordham University's Lincoln Center campus. Dr. Hsu was also co-founder of Fordham's M.S. degree programs in Cybersecurity (2014) and in Data Analytics (2016). He has been director of the Fordham Laboratory of Informatics and Data Mining since 2005.Dr. Hsu has served as program/conference/steering committee co-chairs and co-organizer of many international conferences including DIMACS workshops (1994, 1998, 1999, 2001, 2012, and 2013) and I-SPAN (1st I-SPAN'1994 in Kanazawa, Japan in 1994. The 15th I-SPAN'2019 will be held in Naples, Italy , Sept. 16-19, 2019). He has served on editorial board of many journals and is currently on the editorial boards of: Journal of Interconnection Networks (JOIN), Journal of Advanced Mathematics and Applications, Health Information Science Book Series, International Journal of Big Data Intelligence, Cyber Security: A Peer-Reviewed Journal, and IEEE System Journal (ISJ).
Dr. Hsu has authored/edited 40 books and book chapters, and published over 200 technical papers and has given over 400 talks in conferences and institutions around the world. Dr. Hsu received a Distinguished Teaching Award from Fordham University (2001), an IBM Faculty Award (2012) and three best paper awards from IEEE-AINA Conference (2005), Brain and Health Informatics Conference (2013) in Maebashi, Japan, and the 11th Inter. Conf. on the Frontier of Computer Science and Technology (2017) in Exeter, UK.
He was elected Fellow by ICA (1990), NYAS (1999), and ICIC (2012) and is a Senior Member of IEEE. He is chair of the New York Chapter of the IEEE CIS and a member of the Executive Committee of IEEE New York section.
-
Dr. Hsu's research interests include data science, macro-informatics, cognitive computing, interconnection networks, AI, and combinatorial fusion analysis(CFA). His group has been exploring various aspects of combinatorial fusion including its
architecture, algorithms, and applications since 2002 when it was proposed in a DIMACS Technical Report (Hsu, Shapiro & Taksa; 2002-58 (2002), p.1-47). Methods and practices of CFA have been used in a variety of fields in science, technology, and society. These include (among others): biomedical informatics and cheminformatics, brain science and computational social science, cognitive neuroscience and decision making, information technology and wireless networking, financial informatics and business intelligence, machine learning and multi-layer combinatorial fusion.Among the results achieved by Dr. Hsu's group in the Laboratory of Informatics and Data Mining (LIDM) are: ChIP-seq peak detection, target tracking with occlusion, consensus scoring in virtual screening, stress identification, wireless network selection, market segmentation, portfolio management, intrusion detection, gaze preference prediction using eye movement, and joint decision making.
One of the current research focuses is combinatorial learning theory (CLT). In particular, we are building the foundation of and framework for Deep Fusion which combines deep learning with combinatorial fusion. The Bubble Sort Cayley graph on the symmetric group of order n is used as the learning space with n factorial nodes where n is the number of data items under consideration. CLT will provide efficient and effective learning algorithms to achieve the goal of each of the domain applications in multiple regression, classification, ensemble methods, and other machine learning techniques.