Kyle Doyle ‘21
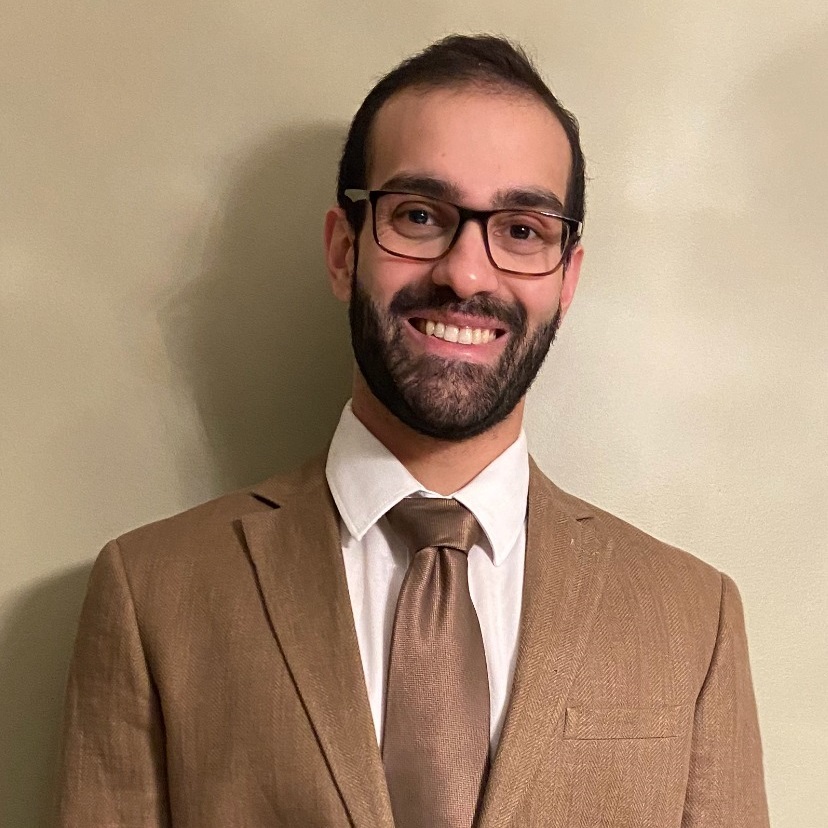
Major: Natural Science
Bio: I am a Natural Science alumnus (FCLC '21) interested in pursuing medical school. As someone interested in matters relating to public health, I was excited to conduct this research from the end of my senior year under the guidance of Dr. Guy Robinson of the Lincoln Center Biology Department. Meanwhile, I just finished my contract as a pandemic response attendant for Lakeridge Health and look forward to continue serving patients conducting clinical research through NYU Langone Health this summer.
Title of Research: Automated Pollen Counting Compared with Traditional Methods for for the 2021 Pollen Season at the Calder Biological Station in Armonk, NY
Mentor: Dr. Guy Robinson, Department of Natural Sciences
Abstract: Public health agencies and the medical community depend on reliable pollen counts to manage seasonal allergies. For over 70 years, Burkard traps have been used to sample air at a fixed rate, followed by light microscopy to evaluate airborne pollen numbers by taxon (type). While generally reliable, the method is time-consuming. Recent efforts to automate the process use electronic/laser counting or image processing algorithms. For the April-October 2021 pollen season in southern New York, we tested the image-processing app, Pollensense, against Burkard data collected at Fordham University’s Louis Calder Biological Station located in Armonk. We recorded counts and produced visualizations using Python and Excel for 15 plant taxa based on numbers, intra- and inter-device, and seasonality data collected over the interval. Overall, the counts showed weak correlation between Burkard and Pollensense’ findings through linear regression (r-square = 0.351). When assessed inter-device, ragweed and amaranth (r = 0.526), mugwort and amaranth (r = 0.566), and ragweed with itself (r = 0.695) were significantly-correlated. When assessed intra-device, 7 and 19 significant correlations were made for Burkard and Pollensense respectively (r > 0.7). Based on seasonality inter-device, ragweed again significantly-correlated with itself (r = 0.621) and 5 and 15 significant correlations were made intra-device for Burkard and Pollensense respectively (r > 0.7). Overall, these data suggest that imaging is not currently a reliable predictor. The stronger intra-device data suggest that sampling algorithms are more consistent, potentially more consistently misidentifying taxa. Development needs to improve to enable this modality to replace traditional counting.